GIS5935 Week 4-- Networks: Network Analysis
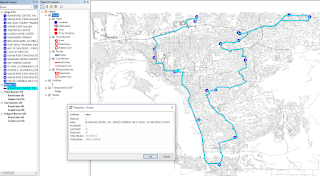
This week in Special Topics in GIS was our first week looking at Networks, we focused on Network Analysis specifically. There were two parts to the lab, the first was a set of introductory exercises to help us refresh on how to create a Network using ArcMap's Network Analyst extension tools as well as review the elements that make up a functioning Network. For the second part of the lab we modified data for provided Network. First I created a new Network Dataset with Turn Restrictions by adding RestrictedTurns and Streets feature classes to the data frame. A point shape file of Facility locations was used for Stops. There were a total of 19 Stops that resulted in a total time of 105.5 Minutes (1.76 hrs). For the final portion of the lab, I created a second Network Dataset which included a Traffic Model. The Traffic model is enabled in the Network setup wizard and allows your network to use historic traffic data for an analysis. The map below displays the new route and...