GIS5100 Week6: Homeland Security--Prepare Minimum Essential Data Sets
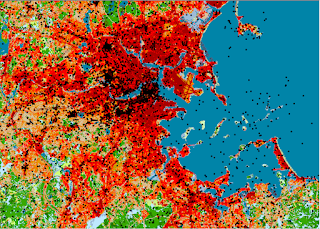
This week we were introduced to MEDS, which stands for Minimum Essential Data Sets. It is a series of minimal essential data sets are critical to the success of a HLS operation. It consists of the following 8 minimal data sets: Ortho imagery Elevation Hydrography Transportation Boundaries Structures Land cover Geographic Names Due to the data used being critical to safety operations, there are minimal goals for resolution, accuracy, and currency in place for both Urban and Large areas. For our lab this week, I prepared a MEDS GIS that helps identify key data sets to prepare for a Boston Marathon bombing crisis scenario. Started by organizing a new map based on a Boston Geodatabase. Then created 7 different group layers to include ortho imagery, DEMs, geographic names, transportation, hydrology, boundaries, structures, and land cover data. Given a specific study area helped identify only the Boston Counties affected along with geographic sites. The transpor